Treating refractory pain through a novel approach of personalised, predictive electrophysiological modelling
EANS Academy. Deli A. 10/06/21; 339530; EP08026
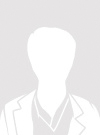
Alceste Deli
Contributions
Contributions
Abstract
Background: Patterns of oscillatory activity associated with pain have been investigated in imaging and electrophysiological studies (animal as well as human). However pain neuromodulation in most centres is based on a trial-and-error process of programming different paradigms. Deep brain stimulation for pain offers direct access to neurophysiological recordings from key nociceptive hubs, therefore additional feasibility for treatment that targets aberrant network activity.
Methods: Our electrophysiological protocol was deployed for a gentleman with a 30-year history of chronic upper limb pain, who had failed numerous surgical and non-surgical pain interventions. We measured activity from sixteen deep brain contacts during the absence of pain and pain induction. We proceeded to compare connectivity matrices, identifying patterns and frequencies associated with high pain state. During one clinic visit, we tested if the areas highlighted by our analyses elicited pain at the predicted nociceptive frequencies. We then programmed stimulation, targeting contacts most associated with pathology and evaluated pain scores longitudinally to assess response.
Results: We identified three areas of four contacts, involved in two different patterns of interaction. Pain-related information flow seemed to change at an individual contact level (see fig. 1 for low gamma activity, p=0.000). After configuring lead stimulation in a frequency that was not involved in nociception during a single clinic visit, pain scores reduced by 50% compared to sham trials.
Conclusion: We devised and successfully deployed a personalized approach of programming neuromodulation parametres, based on functional pain connectivity. In refractory pain this may decrease repeated visits, augment cost-effectiveness and patient satisfaction.
Methods: Our electrophysiological protocol was deployed for a gentleman with a 30-year history of chronic upper limb pain, who had failed numerous surgical and non-surgical pain interventions. We measured activity from sixteen deep brain contacts during the absence of pain and pain induction. We proceeded to compare connectivity matrices, identifying patterns and frequencies associated with high pain state. During one clinic visit, we tested if the areas highlighted by our analyses elicited pain at the predicted nociceptive frequencies. We then programmed stimulation, targeting contacts most associated with pathology and evaluated pain scores longitudinally to assess response.
Results: We identified three areas of four contacts, involved in two different patterns of interaction. Pain-related information flow seemed to change at an individual contact level (see fig. 1 for low gamma activity, p=0.000). After configuring lead stimulation in a frequency that was not involved in nociception during a single clinic visit, pain scores reduced by 50% compared to sham trials.
Conclusion: We devised and successfully deployed a personalized approach of programming neuromodulation parametres, based on functional pain connectivity. In refractory pain this may decrease repeated visits, augment cost-effectiveness and patient satisfaction.
Background: Patterns of oscillatory activity associated with pain have been investigated in imaging and electrophysiological studies (animal as well as human). However pain neuromodulation in most centres is based on a trial-and-error process of programming different paradigms. Deep brain stimulation for pain offers direct access to neurophysiological recordings from key nociceptive hubs, therefore additional feasibility for treatment that targets aberrant network activity.
Methods: Our electrophysiological protocol was deployed for a gentleman with a 30-year history of chronic upper limb pain, who had failed numerous surgical and non-surgical pain interventions. We measured activity from sixteen deep brain contacts during the absence of pain and pain induction. We proceeded to compare connectivity matrices, identifying patterns and frequencies associated with high pain state. During one clinic visit, we tested if the areas highlighted by our analyses elicited pain at the predicted nociceptive frequencies. We then programmed stimulation, targeting contacts most associated with pathology and evaluated pain scores longitudinally to assess response.
Results: We identified three areas of four contacts, involved in two different patterns of interaction. Pain-related information flow seemed to change at an individual contact level (see fig. 1 for low gamma activity, p=0.000). After configuring lead stimulation in a frequency that was not involved in nociception during a single clinic visit, pain scores reduced by 50% compared to sham trials.
Conclusion: We devised and successfully deployed a personalized approach of programming neuromodulation parametres, based on functional pain connectivity. In refractory pain this may decrease repeated visits, augment cost-effectiveness and patient satisfaction.
Methods: Our electrophysiological protocol was deployed for a gentleman with a 30-year history of chronic upper limb pain, who had failed numerous surgical and non-surgical pain interventions. We measured activity from sixteen deep brain contacts during the absence of pain and pain induction. We proceeded to compare connectivity matrices, identifying patterns and frequencies associated with high pain state. During one clinic visit, we tested if the areas highlighted by our analyses elicited pain at the predicted nociceptive frequencies. We then programmed stimulation, targeting contacts most associated with pathology and evaluated pain scores longitudinally to assess response.
Results: We identified three areas of four contacts, involved in two different patterns of interaction. Pain-related information flow seemed to change at an individual contact level (see fig. 1 for low gamma activity, p=0.000). After configuring lead stimulation in a frequency that was not involved in nociception during a single clinic visit, pain scores reduced by 50% compared to sham trials.
Conclusion: We devised and successfully deployed a personalized approach of programming neuromodulation parametres, based on functional pain connectivity. In refractory pain this may decrease repeated visits, augment cost-effectiveness and patient satisfaction.
{{ help_message }}
{{filter}}